Tiny Matrix Extension using RISC-V Custom Instructions
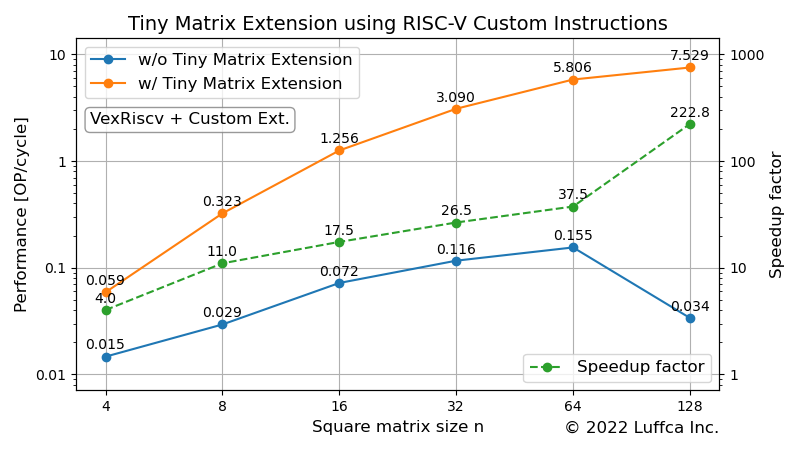
Luffcaでは、RISC-Vのカスタム命令を用いて行列積演算を高速化するプロセッサを開発し、FPGAボードに実装して性能を評価しました。
関連記事は、こちら。
- Matrix Multiplication based on the RISC-V Vector Extension
- Tiny Matrix Extension using RISC-V Custom Instructions(本記事)
- Applying the Tiny Matrix Extension to ML Inference
RISC-V Custom Instructions
RISC-Vでは、カスタム命令用の命令コード枠が仕様に定められた拡張であるMAFDCV等の命令コードと重複しないように予め確保されており、カスタム命令の追加によるカスタム拡張を実施できます。これによって、特定領域に特化して設計されたプロセッサであるDSA(Domain Specific Architecture)の開発も可能になります。
UCB(カリフォルニア大学バークレイ校)で開発されているDNNアクセラレータのGemminiでは、Rocket等のプロセッサがRoCC(Rocket Custom Co-processor)インターフェースを介してアクセラレータと接続され、カスタム命令を用いてアクセラレータを制御しています。
また、Building an ML Processor using CFU Playground (Part 1)等の記事で紹介したML(機械学習)プロセッサは、CFU(Custom Function Unit)インターフェースを介してVexRiscvプロセッサとSIMD MAC(multiply-accumulate)命令用の回路を接続し、カスタム命令のSIMD MACを用いてMLモデルの推論を高速化しています。
Tiny Matrix Extension
この記事におけるTiny Matrix Extensionは、V(ベクトル)拡張のようにRISC-VのISA仕様に定められている拡張ではなく、RISC-Vのカスタム命令を用いた行列積のカスタム拡張です。
Tiny Matrix Extensionの目的は、省リソースで以下の行列積コードを高速化することです。
// C = AB with A = [n x n], B = [n x n], C = [n x n] void matmul_int8(int32_t* c, const int8_t* a, const int8_t* b, const int n) { for (int i = 0; i < n; ++i) { for (int j = 0; j < n; ++j) { int32_t sum = 0; for (int k = 0; k < n; ++k) { sum += a[i * n + k] * b[k * n + j]; } c[i * n + j] = sum; } } }
なお、機械学習への応用を考慮し、行列AとBの成分はint8_t
、行列Cの成分はint32_t
としています。
前回の記事では、ソフトウェア開発として、RISC-Vのベクトル拡張(RVV)仕様に基づく行列積カーネルを作成し、RTLシミュレータを用いて性能を評価しました。
ここでは、ハードウェア/ソフトウェア協調開発を実施し、FPGAボードに実装して性能を評価しています。
RISC-V Processor with Tiny Matrix Extension
FPGAボード、カスタムプロセッサ及びカスタム拡張であるTiny Matrix Extensionの主な仕様は、以下の通りです。
- FPGAボード: Digilent Arty A7-35T
- FPGA: XC7A35T
- DRAM: 256 MB
- カスタムプロセッサ: 32-bit RISC-V
- 基となるプロセッサ: VexRiscv
- ISA: RV32IM + Tiny Matrix Extension
- 動作周波数: 100 MHz
- Tiny Matrix Extension
- ハードウェア・インターフェース: CFU
- ソフトウェア・インターフェース: カスタム命令
- 正方行列のサイズn: 4の倍数で128以下
- 入力データ:
int8_t
- 出力データ:
int32_t
アイキャッチ画像と下の表が、Tiny Matrix Extensionの性能を表しています。Performance [OP/cycle]は、10回のプログラム実行の平均(Mean)を用いて算出しています。
Square matrix size n |
Cycles | Performance [OP/cycle] |
Speedup factor |
|
---|---|---|---|---|
Mean | Stdev | |||
16 | 6522 | 244 | 1.256 | 17.5 |
32 | 21211 | 726 | 3.090 | 26.5 |
64 | 90297 | 420 | 5.806 | 37.5 |
128 | 557096 | 243 | 7.529 | 222.8 |
以下は、プログラム実行時のコンソール出力の一例を示しています。
... ---------------------------------------------------------------- Calculating a (32 x 32) x (32 x 32) matrix multiplication....... ---------------------------------------------------------------- Initializing matrices... Calculating imatmul with tiny matrix extension... The execution took 21047 cycles. The performance is 3.113 OP/cycle. Calculating imatmul without tiny matrix extension... The execution took 562829 cycles. The performance is 0.116 OP/cycle. Verifying result... Passed. ---------------------------------------------------------------- Calculating a (64 x 64) x (64 x 64) matrix multiplication....... ---------------------------------------------------------------- Initializing matrices... Calculating imatmul with tiny matrix extension... The execution took 90492 cycles. The performance is 5.793 OP/cycle. Calculating imatmul without tiny matrix extension... The execution took 3384030 cycles. The performance is 0.154 OP/cycle. Verifying result... Passed. ...
まとめ
Luffcaでは、RISC-Vのカスタム命令を用いて行列積演算を高速化するプロセッサを開発し、Digilent社のArty A7-35Tに実装して性能を評価しました。正方行列のサイズnが32と64の場合、Tiny Matrix Extensionなしの場合と比較して、それぞれ26倍と37倍のスピードアップを実現しました。